Data-Driven AI transformation in Healthcare
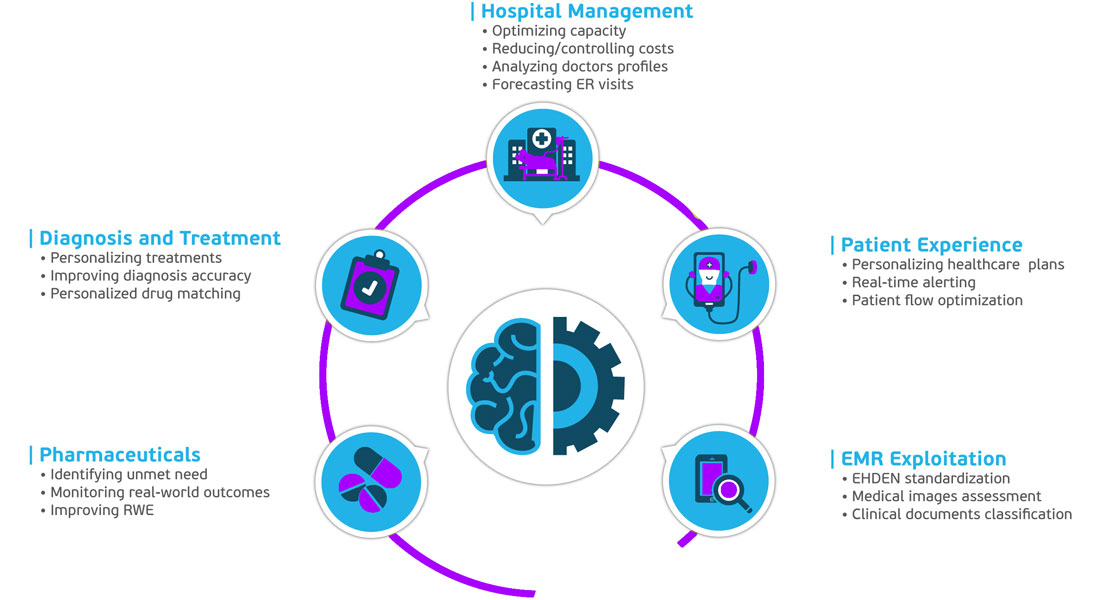
Among others, Covariance Team enables healthcare organizations:
- To unlock the power of health real-world-data to fuel innovation in health and improve patient care is at the heart of healthcare revolution. This is made possible by advances in health technologies and achieved through the extraction of real-world evidence hidden in patients’ medical records.
- To foster research, maximize their operational efficiency and minimize costs, by analyzing large, complex datasets with advanced analytics and machine learning, and the integration of real-time analysis into existing systems and processes.
- To improve customer satisfaction, operational efficacy, financial and administrative performance, increasing revenue and ROI, optimizing resources, and advancing medical research capabilities such as personalized therapies, while in parallel introduces clients to the market of real-world-data, a multibillion market.
- In pharma to identify unmet therapeutic needs, improving RCTs design, accelerating time to market, refining commercial strategies, achieving label expansion, and building clinical-decisions-support systems, while on parallel guide them to their quest in identifying real-world-data sources.
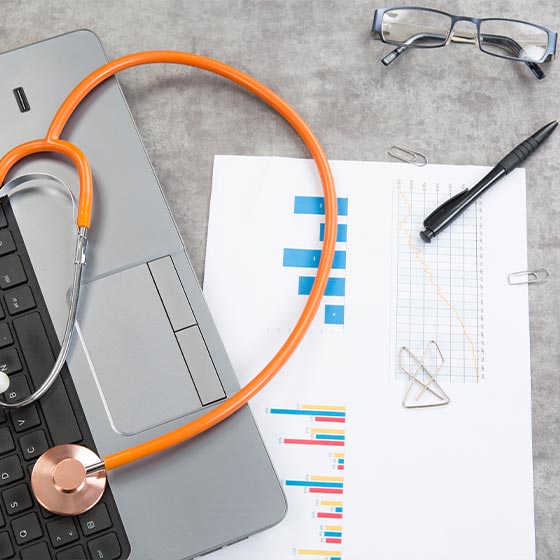
Personalized Risk Estimation (Probability Assessment of future Lab test outside of normal limits)
Goal
Personalized Medicine
Early Intervention
Data Driven Decision Making
Long-term Health Management
Challenges
1. By predicting potential abnormalities, conditions can be identified before symptoms appear or become severe, allowing for earlier diagnosis and treatment.
2. Traditional healthcare systems often react to existing conditions. Predictive models enable a proactive approach, focusing on prevention and early intervention.
3. Early intervention can prevent the development of more serious conditions, which are often more expensive to treat, thereby reducing overall healthcare costs.
4. Predictive models support personalized medicine by identifying individual risks and enabling tailored treatment plans, improving patient outcomes.
Opportunities
Personalized treatment
Advancements in Medical Research
Innovation in Healthcare Technology
Cost Savings by reduction in hospital readmissions
Public Health Surveillance
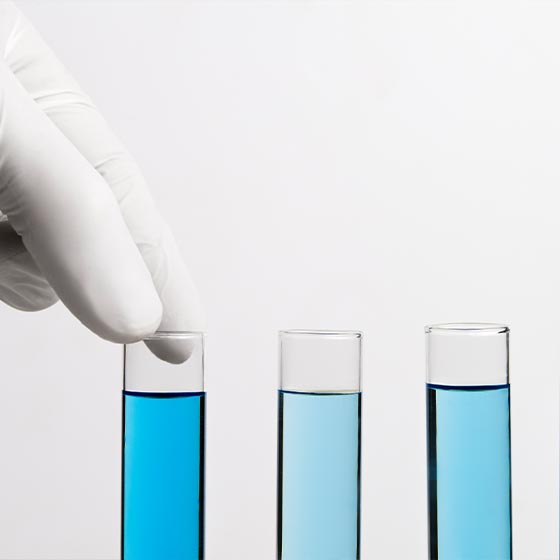
Probability of illness (disorder risk assignment throughout lab test)
Goal
Early Detection and Diagnosis
Clinical Decision Support
Resource Optimization
Accuracy and Consistency
Challenges
1. Medical data, including lab tests, are vast and complex, with various formats and sources. ML models can process and analyze large datasets quickly, identifying patterns and correlations that might be missed by human analysis.
2. Human error and variability in interpreting lab results can lead to misdiagnoses which can be minimized by the supportive AI tools the likelihood of diagnostic errors.
3. The model can detect early signs of illness from lab results, facilitating early intervention and better patient outcomes.
4. Complex cases require the integration of multiple data points and expert knowledge. models support clinical decision-making by integrating various data sources and providing comprehensive risk assessments.
Opportunities
Enhanced Predictive Analytics: Enable proactive healthcare measures and preventative strategies.
Tailor treatments to individual patients based on their specific risk profiles
Increase the likelihood of early detection, leading to timely and more effective treatments.
Reduce morbidity and mortality rates by identifying high-risk patients sooner.
Allocate medical resources more effectively by prioritizing patients based on their risk levels.
Reduce healthcare costs by minimizing unnecessary tests and procedures.
Support clinicians with data-driven insights, enhancing decision-making capabilities.
Monitor population health trends and detect outbreaks earlier through analysis of aggregated lab test data.
Integration of Multimodal Data: Combine lab test results with other types of medical data (e.g., imaging, genetic information) for a more comprehensive health assessment.
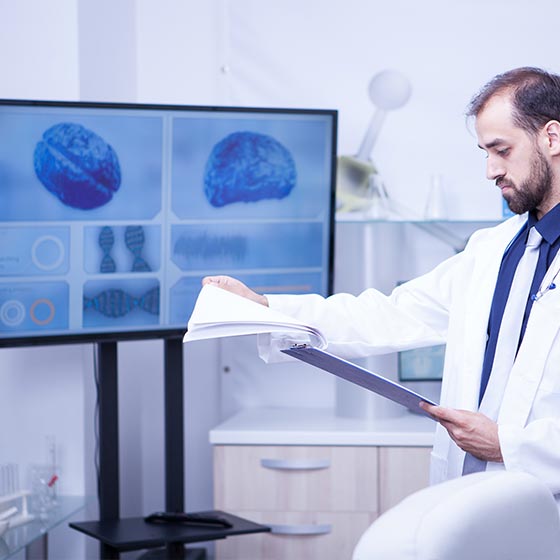
Imaging Test Analysis
Goal
Early Detection and Diagnosis
Improved Accuracy
Efficiency and Speed
Augmentation of Clinical Decision-Making
Challenges
1. Models can analyze complex patterns and subtle features within medical images that may not be easily detectable by human observers.
2. These models can serve as decision support tools for clinicians, offering second opinions or highlighting areas of concern in medical images.
3. In regions with limited access to specialized medical professionals, models can provide access to advanced diagnostic capabilities without the need for physical presence of specialists, thereby improving healthcare access and equity.
Opportunities
Automation of image analysis tasks can streamline workflow in radiology departments, allowing radiologists to focus more on complex cases and patient care, thereby improving overall efficiency in healthcare delivery.
Faster and more accurate diagnosis can lead to quicker treatment decisions and improved patient management, enhancing the overall quality of patient care.
Models can serve as decision support tools, providing insights and recommendations to clinicians, thereby aiding in clinical decision-making and potentially reducing diagnostic variability.
By improving diagnostic efficiency and reducing unnecessary tests or delays in diagnosis, models can potentially lead to cost savings for healthcare systems and patients alike.
The development of such models for medical imaging drives technological innovation in AI, computer vision, and healthcare informatics, pushing the boundaries of what is possible in medical diagnostics and patient care.
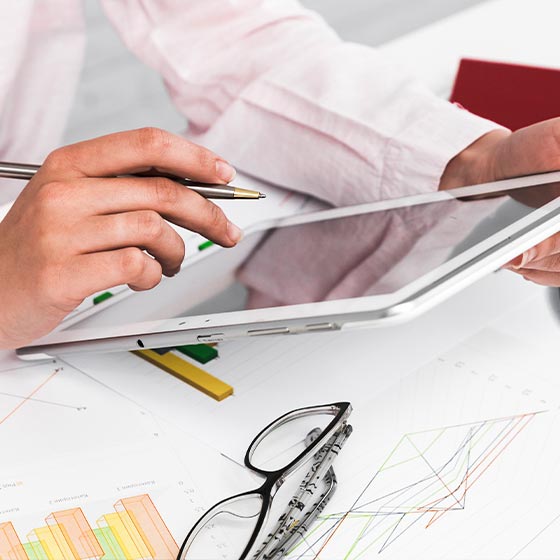
Unstructured Text Analytics
Goal
Automated Analysis
Monitoring health trends
Change Point detection
Efficiency
Challenges
1. NLP models can assist in improving diagnostic accuracy by identifying subtle patterns or changes in imaging results that human eyes might miss. This can lead to earlier detection of diseases and more accurate diagnoses.
2. NLP systems can help standardize the interpretation of medical imaging reports across healthcare providers and institutions, reducing variability and ensuring consistency in care delivery.
3. By monitoring changes in health status over time through automated analysis of imaging data, NLP systems can facilitate early detection of disease progression or treatment effectiveness, enabling timely interventions.
4. Providing decision support by highlighting critical findings or changes in imaging results can assist healthcare providers in prioritizing cases and making informed treatment decisions more efficiently.
Opportunities
Enhanced Diagnostic Capabilities: NLP can assist healthcare providers in detecting subtle patterns and changes in imaging data that may not be immediately apparent to human observers. This can lead to earlier and more accurate diagnoses of diseases and conditions.
Personalized Medicine: By analyzing large volumes of imaging data and patient records, NLP systems can help identify biomarkers or specific characteristics that indicate a patient's response to treatment. This enables personalized treatment plans tailored to individual patient needs.
Predictive Analytics: NLP-powered systems can analyze trends and patterns in imaging data over time, providing insights into disease progression, treatment outcomes, and potential complications. This supports predictive analytics for better patient management and preventive care.
Workflow Optimization: Automating the analysis of imaging reports through NLP can streamline clinical workflows, reduce administrative burden, and optimize resource allocation in healthcare settings.
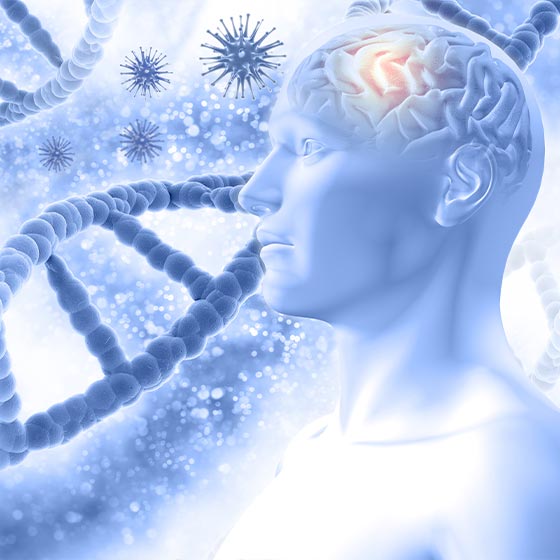
Parkinson Screening Application
Goal
Early Detection
Cost-Effectiveness
Automation
User-Friendly Interface
Challenges
1. Parkinson's disease manifests differently in everyone. The app can account for the variability in symptoms such as tremors, bradykinesia (slowness of movement), rigidity, and postural instability.
2. The app can accurately distinguish between movements characteristic of Parkinson's disease and those that are not, while minimizing false positives and false negatives, is crucial for its clinical utility.
Opportunities
Automatic Patient Monitoring & Continuous monitoring
Telehealth & Digital Experience
Automatic Alert System
Personalized Monitoring
No need for transportation and waiting
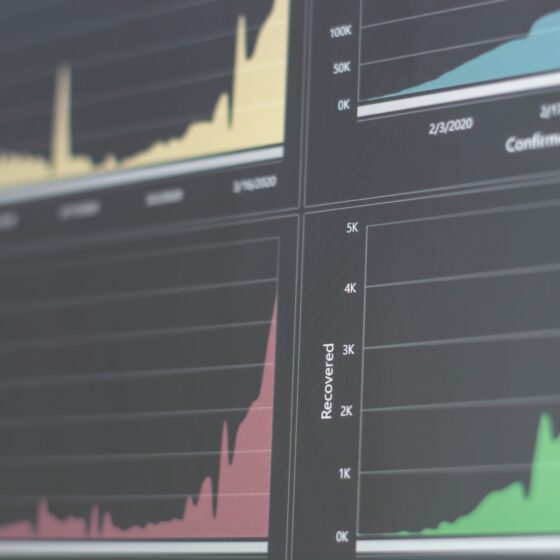
Real World Data
Goal
Strategic Advantage
Better/Personalized Treatments
Customer Satisfaction
Increased Revenue and ROI
Challenges
Increased regulation and competition restricts the success rate of Technology Assessment, and reduces Market Access prices.
Opportunities
Real world evidence significantly increases the success rates for drug market access and costs less that clinical trial evidence. Covariance can provide valuable insights from real world heterogenous data to strengthen a new drug case.
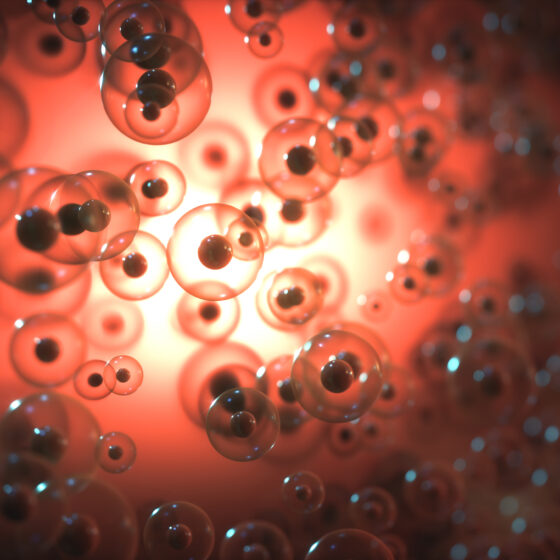
Large-Scale HDCN (Human disease complex networks)
Goal
Strategic Advantage
Better/Personalized Treatments
Customer Satisfaction
Increased Revenue and ROI
Challenges
The vast majority of DNA tests link a certain disease to one or two specific genes. On the contrary complex diseases are known to be associated with a variety of genes, of which some are linked to other disease respectively. It is therefore sought to map all known associations and their correlation in order to achieve more accuracy in genomic analysis.
Opportunities
AI based analysis of disease-networks in a large-scale perspective
- Extraction of phenotypical knowledge from multiple sources using text mining/NLP.
-Identification and use of well-known biological databases.
-Creation of large disease-networks. Mapping and analysis of common features for drug repurposing.
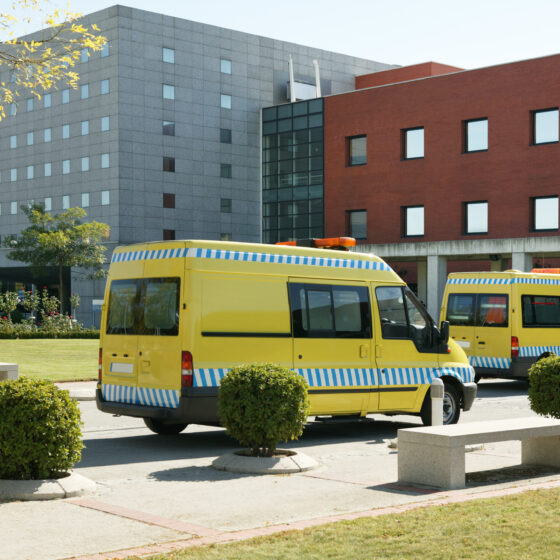
Predicting Hospital Readmissions (Preventing Readmissions with Data Analytics and AI)
Goal
Strategic Advantage
Better/Personalized Treatments
Customer Satisfaction
Increased Revenue and ROI
Challenges
Patients with serious and chronic diseases are hospitalized for a period and then being discharged. Unfortunately, multiple studies have shown that up to 25% of these patients will be readmitted within 30 days to be treated again, in some cases with less favorable outcomes. Focusing on value-based care, healthcare providers try to prevent unnecessary readmissions and improve patient care outcomes. Readmission can be significantly reduced by taking steps while the patient is still in the hospital, defining different actions during discharge, and taking steps post discharge to ensure compliance with home care regimens.
Opportunities
AI is ideally adapted in tasks where data inputs are rather complicated and may elude clinicians. In projecting Readmissions risk data are required about the specific patient’s recent care, their current condition, treatment, their home life and other risk factors from patients’ electronic medical records. AI models use this information to provide preemptive assessment of their risk and notify clinicians while the patient is still hospitalized. AI provides reasons that might lead to readmission and provide recommendations for the most likely types of successful treatments according to a patient’s history. The reason codes are valuable to clinicians because they can pinpoint precise areas to focus on when developing a care plan for the patient, thus preventing unnecessary and costly tests.
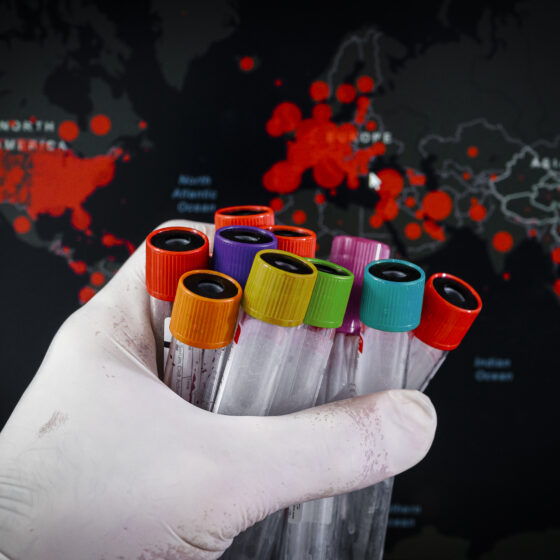
Medical Testing (Predicting Medical Test Results with Data Analytics and AI)
Goal
Strategic Advantage
Better/Personalized Treatments
Customer Satisfaction
Increased Revenue and ROI
Challenges
According to the National Academy of Medicine, waste in healthcare is widespread and is estimated to be about $765 billion annually. One of the key areas of waste is unneeded testing or routine tests that are rarely necessary. As stated in ResearchAndMarkets, the global laboratory services market is expected to grow at a compound annual growth rate of 4.21% over the forecast period (2020 -2025), to reach a market size of US$468.249 billion in 2025 from US$365.690 billion in 2019. The number of unnecessary tests result in significant costs that do not necessarily contribute essentially to healthcare quality or positive patient outcomes.
Opportunities
Using Advanced Data Analytics and AI we help clinicians make better decisions by defining the types of tests that are likely to be useful for a patient. One of the advantages of AI is its unique ability to integrate large volumes of data and identify patterns that may be subtle or difficult for humans to recognize. These subtle patterns have an huge potential to alert clinicians to important physiologic changes that need to be addressed. With an AI-driven application we can provide indications of which tests are likely to produce definitive or valuable results based on the patient’s medical history and current symptoms. With this knowledge, the clinician can advise optimal treatments with the best outcomes and minimize the number of tests, which saves time and reduces costs to the patient.
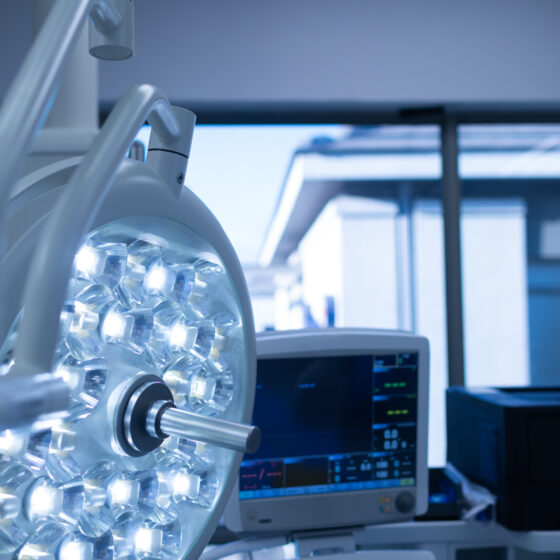
Predicting ICU Transfers (Saving Lives by Catching Patients Before the Crash with Data Analytics and AI)
Goal
Strategic Advantage
Better/Personalized Treatments
Customer Satisfaction
Increased Revenue and ROI
Challenges
Studies show that patients who undergo an unplanned transfer to the ICU experience worse outcomes than patients admitted directly. These patients typically stay in the hospital 8 to 12 days longer and have significantly higher mortality rates – these patients account for only 5% of patients but represent one-fifth of all hospital deaths. The challenge is to find patients before they “crash” and need to be moved to the ICU, but these patients often lack recognizable symptoms that clinicians can access as ones leading to a serious change in condition.
Opportunities
AI models can be used to find patients who are likely to crash. The machine learning models use patient medical records, laboratory results, and vital signs from patients to find early warning signals of deteriorating condition. These models can be used with existing patients in real-time to determine their risk of a crash and as part of an early warning system for clinicians so they can intervene before the ICU transfer is needed. The AI system can also provide reason codes for a specific patient, which can be a helpful tool to clinicians to understand where they should begin their treatment.
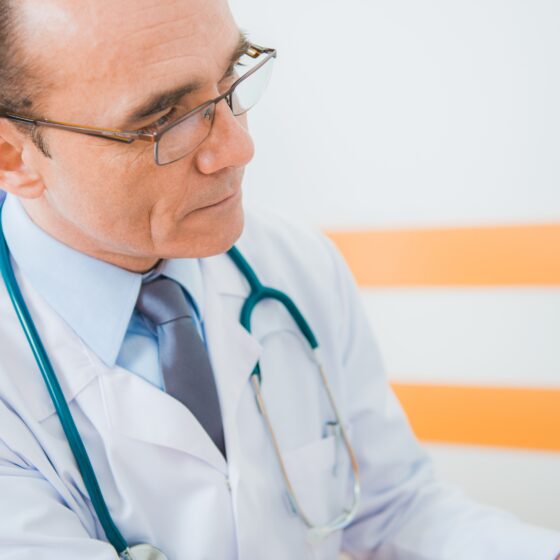
Physician Profiling (Understand physician profiles and clinical performance)
Goal
Strategic Advantage
Physician Assessment
Customer Satisfaction
Increased Revenue and ROI
Challenges
Cost reduction
Quality of services improvement
Optimize staff performance
Opportunities
The economic evaluation & qualitative assessment of a physician’s profile is a measure of quality of Healthcare Service. By using indicators (KPIs) measuring the Physician’s performance in a Healthcare unit we can reduce healthcare costs, improve quality and increase patient satisfaction simultaneously . The AI system can develop a system for KPIs evaluation and desing the necessary intervention steps.
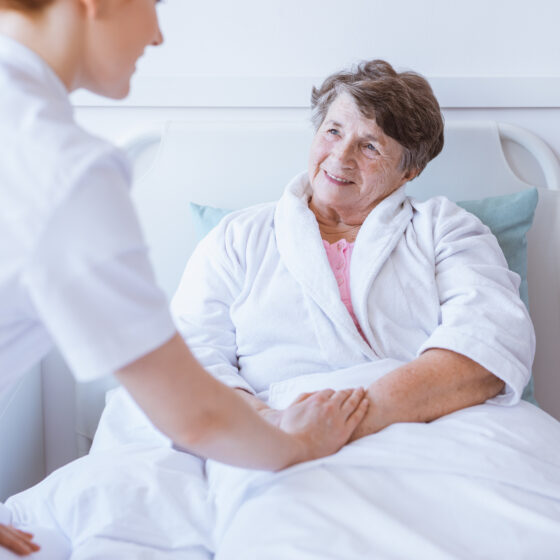
Patient Profiling (Optimization of healthcare services with personalized care management interventions)
Goal
Strategic Advantage
Better/Personalized Treatments
Customer Satisfaction
Increased Revenue and ROI
Challenges
Patient Evaluation
Cost reduction
Quality of services improvement
Opportunities
Analyze available data, such as:
-Medical services consumer profile
-Geolocation
-Connection with physicians
-Satisfaction from the provided services
-Needs & Preferences
-Optimization of catchment area
-Potentially suboptimal spatial health accessibility of patients.
-Maximization of patient value
-Identify the economic impact of patient navigation in the Healthcare unit to increase revenue per patient through efficient cross, deep & up selling activities.
-Patient Understanding
-Development of innovative products & services based on patient understanding. Using VOC surveys as a tool to reveal customer’s feedback and improve decision making that contribute to a positive return on investment.
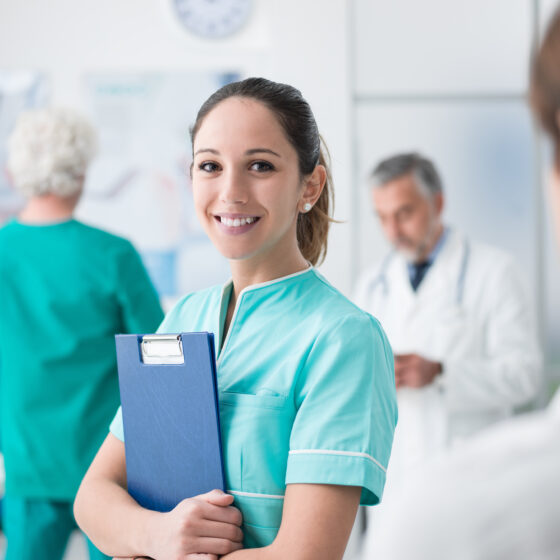
Optimization of resources (Optimization of healthcare services with personalized care management interventions)
Goal
Strategic Advantage
Better/Personalized Treatments
Customer Satisfaction
Increased Revenue and ROI
Challenges
Improve utilization
Cost reduction
Operational efficiency & effectiveness of human & asset capital
Optimize supply chain
Optimize the network between the healthcare units & supplies
Opportunities
Analyze all available data such as:
-Staff utilization data
-Asset utilization data
-Suppliers data
-Products usage characteristics
-Products quality
-Products costs